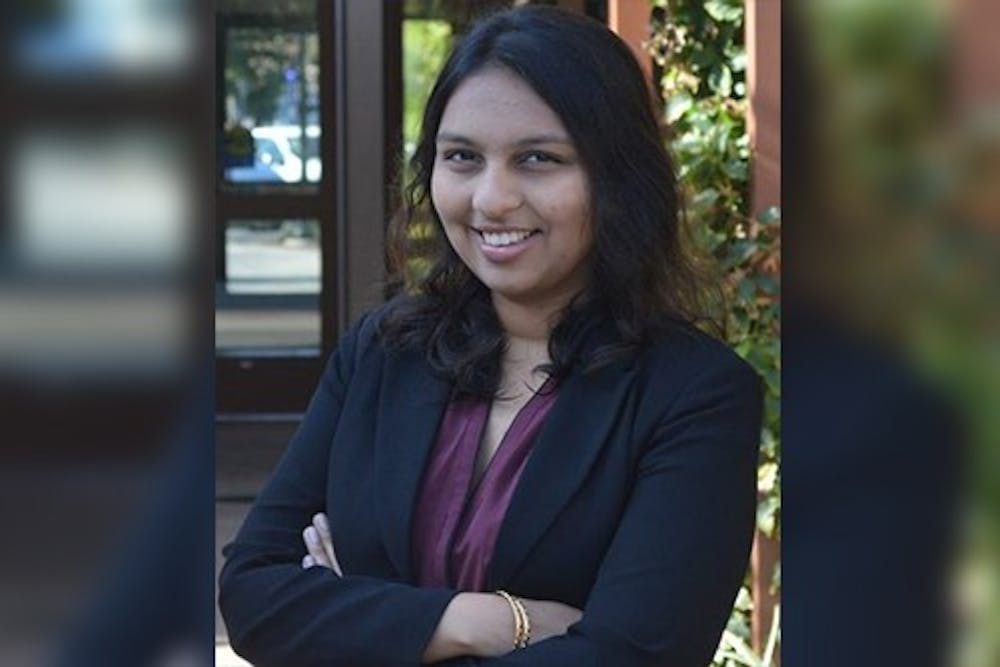
A study by a Penn Assistant Professor of Operations, Information, and Decisions Hamsa Bastani discovered that the application of machine learning made COVID-19 screening at Greece’s border more efficient (Photo from School of Engineering and Applied Science).
A study by a Penn researcher discovered that the application of machine learning made COVID-19 screening at Greece’s border more efficient.
Penn Assistant Professor of Operations, Information, and Decisions Hamsa Bastani collaborated with Greek researchers during the summer of 2020 to evaluate the effectiveness of Eva, a machine learning tool designed to track data on travelers’ COVID-19 screening results along with demographic information and region of origin, Penn's Leonard Davis Institute of Health Economics reported.
Tracking this information enabled Eva to identify areas of immediate concern for COVID-19. According to the study, officials targeted individuals from these areas for random testing and quarantine mandates at a time when rapid testing was not very accessible in Greece.
Eva refines its algorithm and predictions to be more accurate by collecting data over time. The study reported that the reinforcement learning system allowed Greek border officials to identify up to four times as many asymptomatic COVID-19 cases compared to random testing strategies utilized in other countries.
Researchers of the study believe that Eva also financially boosted the Greek government by reducing the number of tests that would have been required to effectively mitigate transmission risk via random sampling by up to 85 percent, LDI reported.
The study compared the effectiveness of machine learning strategies in identifying travelers who should be prioritized for testing to the effectiveness of more traditional data analysis methods such as comparing the average per capita case, hospitalization, and death rates of different locales.
Data from the study demonstrated that Eva was up to 1.45 times more effective in detecting asymptomatic coronavirus cases compared to the more traditional approaches.
Researchers of the study made Eva's code open source so that machine learning could become a more commonplace tool in border screening strategies and so that countries can adapt the software to their own needs and circumstances, LDI reported.
Bastani and the other researchers involved in this project aim to improve COVID-19 screening measures implemented at borders around the world in order to reduce international transmission rates and mitigate the risks associated with travel during a pandemic, LDI reported.
The Daily Pennsylvanian is an independent, student-run newspaper. Please consider making a donation to support the coverage that shapes the University. Your generosity ensures a future of strong journalism at Penn.
Donate